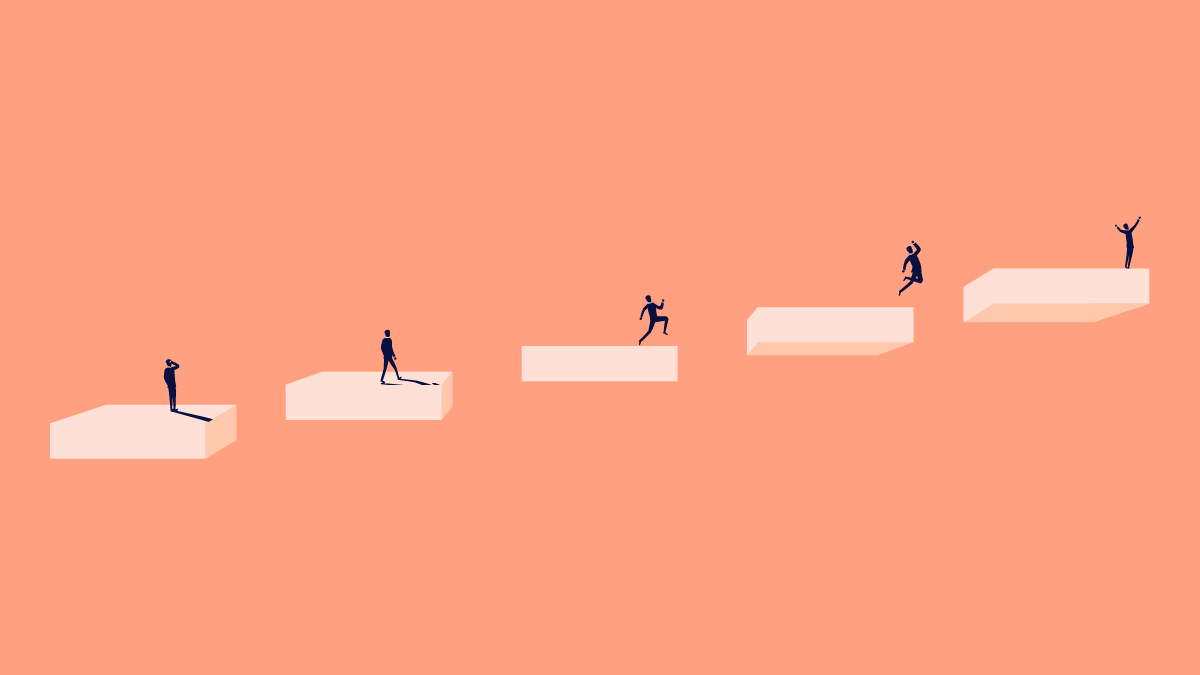
As the life sciences are digitalizing at an unprecedented scale and rate, data lifecycle management is becoming more important than ever.
Nearly every team member handles data in some capacity, where well-managed, accurate data can make all the difference in regulatory compliance, research breakthroughs, and patient safety.
And now with the increased focus on data integrity regulations and the rise of Industry and Quality Assurance 5.0, where human expertise and cutting-edge technology work hand in hand, implementing best practices for proper data lifecycle management is a must.
But what exactly is it, what are the eight stages of data lifecycle management and why should you care? Let's break it down!
What is data lifecycle management?
Simply put, data lifecycle management is the process of handling data from creation to disposal in a structured, secure, and efficient way.
In life sciences, where data fuels everything from drug development to clinical trials, data lifecycle management ensures compliance, traceability, and accuracy—all while keeping up with evolving regulations. A well-managed data lifecycle isn't just about organization; it's about making smarter, faster decisions while avoiding risks.
Why understanding the data lifecycle is crucial
Managing data properly isn’t just a “nice-to-have” in the life sciences—it’s a must. Here’s why:
- Regulatory compliance: Strict regulations (think FDA, EMA, or ISO standards) require meticulous data management. Poor data handling can lead to failed audits, fines, or worse.
- Data integrity and security: With sensitive research and patient data at stake, protecting information from loss, corruption, or breaches is non-negotiable.
- Efficiency and cost savings: A structured data lifecycle management strategy reduces duplication, minimizes errors, and ensures data is available when and where you need it.
- Better decision-making: High-quality, well-managed data leads to more accurate insights, driving innovation and success.
Now, let’s dive into the eight key stages of the data lifecycle.
The 8 phases of data lifecycle management
Stage 1: Generation
Data is born! The first step to managing your data lifecycle successfully is data generation. In the life sciences industry, data generation is an ongoing process resulting from R&D, compliance, and manufacturing records. Some of the important activities that lead to the generation of the data are:
- Raw material specifications
- Raw material inventory
- R&D experiments and manufacturing batch records
- In-process and finished product test results for R&D experiments
- Compliance data of R&D activities
- Batch release data
- Marketing and sales data
- Finance data
Stage 2: Collection
The data generation activities in the first stage of data lifecycle management lead directly to data collection. This may sound simple, but collecting large chunks of data accurately is quite the challenge!
This is where software-driven automation can come into play. A systematically planned data policy may help you manage this step effortlessly. Some of the leading software solutions to collect data are listed below:
Stage 3: Storage
Data needs a home. Now that your data is available in an accessible and usable form, the next step in the data lifecycle management is to ensure that it is stored safely for future use.
Depending on your organization's IT policies, you can store your data using off-site cloud services such as Data Historian Platform, or on-site options like SSDs, hard disk drives, magnetic LTO tape, or even DVDs. One very important factor for on-site storage methods is to consider their conservatively expected lifespan.
Stage 4: Processing
Once data has been collected and digitalized with the help of your preferred software services, it must be processed. A good data lakehouse can help you achieve this step. Data processing may include – but is not limited to – the following activities:
-
Data wrangling in which data from various software sources is combined in a meaningful way to clean and transform data from raw form into a more accessible and usable format.
-
Data compression is an essential processing step in which data is transformed to be more efficiently stored.
-
Data encryption is another data processing step in which data is translated into another form of code to protect it due to privacy concerns.
-
Data processing may also include the simple act of converting printed data into digital data.
Stage 5: Management
Just storing data is not enough! That’s why this next step in the data lifecycle is to manage all stored data. Backing up your data is one important step to protect data from unintentional damage or loss. Your data backup strategy should address the following questions:
- Who will be responsible for managing data backups?
- Will it be an automated process?
- How frequently will the backup take place?
- How many copies of the backup will be created?
- Where will the backup be stored?
- Who will have access to the backup?
Another important aspect of data management is defining user access levels. This may include particular info about data viewing, editing, analyzing, and deleting rights of users based on their authority level.
“Keep it simple. The lifecycles can be of great level of detail but if you keep them simple, they become manageable. A minor change in daily work shouldn’t require updating 20 documents, you don’t want to be in that situation.”
Kurt In Albon, Global Head of Information Quality at Lonza
Stage 6: Analysis
This step is truly the core of the data lifecycle. Here, data analysts perform various tests on the data to derive meaningful insights.
Your analysts may perform several graphing options on the data, followed by data modeling using machine learning, artificial intelligence, statistical methods, or mathematical methods.
The data analysts will also select the most appropriate data analysis technique based on the problem statement they are trying to solve. This may include performing model validation tests to finalize the model for future predictions based on your data.
Stage 7: Visualization and interpretation
Once you have successfully validated the model in step 6, you can move on to Data Visualization. The purpose of this step is to represent the information contained in the data in a palpable format. Your data analyst may create various data visualization dashboards to help users visualize the data. For example, one data visualization dashboard can highlight manufacturing process differences in the out-of-specification batch vs. the successfully released batch.
Users derive meaningful insights from the charts and predictions displayed on data visualization dashboards. This data interpretation is used for important business decisions and process improvements.
Stage 8: Destruction
It is crucial that data destruction (also known as data purging) also removes every obsolete copy of an item. This is typically done in an archive storage location. The challenge of this phase of the lifecycle is to ensure that all data has been properly destroyed. But even before destroying data, it is important to ensure that the targeted items have exceeded their regulatory minimum retention period.
In the life sciences, companies must retain their data records according to all applicable regulations for the specific products they manufacture. For example, products manufactured in or exported to the USA need to comply with general record-keeping requirements described in § 211.180, meaning records must be retained for at least one year after the expiration date of a batch and for certain over-the-counter (OTC) drugs for at least three years after distribution of the batch (§ 211.180(a)).
Once this period ends and data is no longer required to be retained, that data no longer serves a meaningful purpose to your organization. Therefore, it can be safely removed from storage archives. However, before removing the data, manufacturers should also ensure that – apart from the mandatory record retention period – the targeted data is not being actively used as a benchmark (internal standard) or as calibration data for a machine learning model deployed in real-time quality control procedures for assessing or predicting future outcomes.
For a deeper dive into the data lifecycle and other key concepts in data integrity and data governance, check out the Scilife Academy video below!
Common data lifecycle challenges and how to overcome them
Even with the best intentions, managing data comes with its fair share of roadblocks. Here are some common challenges and ways to tackle them:
- Data silos:
Different departments using separate systems make collaboration difficult.
Solution: Implement centralized digital solutions like Scilife’s eQMS to streamline data access.
- Regulatory compliance struggles:
Constantly changing rules can make compliance feel overwhelming.
Solution: Automated compliance tools help track and maintain audit trails effortlessly.
- Data security risks:
Cyber threats and accidental data loss are real dangers.
Solution: Use robust encryption, access controls, and backup solutions.
- Outdated or inaccurate data:
Bad data leads to bad decisions.
Solution: Regular data audits ensure accuracy and relevance.
Scilife’s eQMS solution helps address all these challenges by offering secure, automated, and regulatory-compliant data management tools for the life sciences industry.
Key takeaways
- Data is the backbone of the life sciences industry—managing it correctly is non-negotiable.
- Managing the data lifecycle effectively isn’t just about compliance, it’s about efficiency, security, and innovation.
- By understanding the eight stages, addressing challenges, and using the right tools, your life sciences organization can maximize the value of its data while staying compliant and secure.
FAQs: Everything you need to know about data lifecycle management
What are the types of data flow in the life sciences?
Data flows in various ways, including structured data (databases, spreadsheets), unstructured data (emails, documents), and real-time streaming data (sensor readings, clinical trial monitoring).
What is a data lifecycle management strategy for the life sciences?
A strategy outlines how data is handled from creation to disposal, ensuring compliance, security, and accessibility while optimizing efficiency.
What are the goals of data lifecycle management?
The primary goals are compliance, security, efficiency, and accuracy, ensuring data integrity throughout its lifecycle.
What are some best practices for data lifecycle management?
Use automation to streamline workflows.
Implement access controls to protect sensitive data.
Regularly audit and clean data to ensure accuracy.
What real-time data processing techniques are used in life sciences?
Common techniques include streaming analytics, edge computing, and AI-driven automation to process lab and clinical trial data instantly.
What data management software could I use?
Solutions like Scilife’s eQMS, cloud-based LIMS (Laboratory Information Management Systems), and regulatory compliance software help streamline data lifecycle management.
Conclusion
Your systematic approach to data lifecycle management is instrumental in driving data-based decisions for your organization. Interlinking and automating these eight steps for effective and efficient data lifecycle management will help you stay focused on the ultimate goal of data interpretation. Data truly is the ‘new oil’ of the 21st Century – and it is up to you to make it the driving force of your organization’s future.
watch our on-demand training webinar with Kurt In Albon, Global Head of Information Quality at Lonza, as he shares strategies for overcoming data governance and lifecycle challenges!